|
Human-end product managers, interaction, testing, R&D, operations, project managers, etc. have all transformed into end-end products. But most View the advantages and limitations of decision trees in detail. Advantages: Easy to understand and explain. The generated decision rules can be directly transformed into business strategies. Ability to handle numeric and categorical data without requiring special preprocessing of the data. Able to handle non-linear relationships and missing values. Limitations: Easy to overfit, especially for complex or noisy data sets. Being sensitive to small changes in input data can result in completely different decision tree generation. Decision trees may be too complex and require pruning and other means to optimize. 2. Random
Overview of the Random Forest Principle of "Trees into a Forest, Wisdom Emerges" Random forest is an ensemble learning method. It is composed of multiple decision trees and their average or voting results are taken as the final prediction. Each decision tree is randomly selected from a subset of samples. r le is trained based on randomly selected Rich People Phone Number List features. This randomness and diversity ensure that the overall prediction accuracy remains stable and powerful even if a single decision tree has deviations. The advantages and improvements of random forests have excellent resistance The over-fitting ability can effectively handle the problem of high-dimensional data and a large number of features. It can evaluate the importance of each feature to help feature selection. It can perform regression and classification tasks with superior performance.
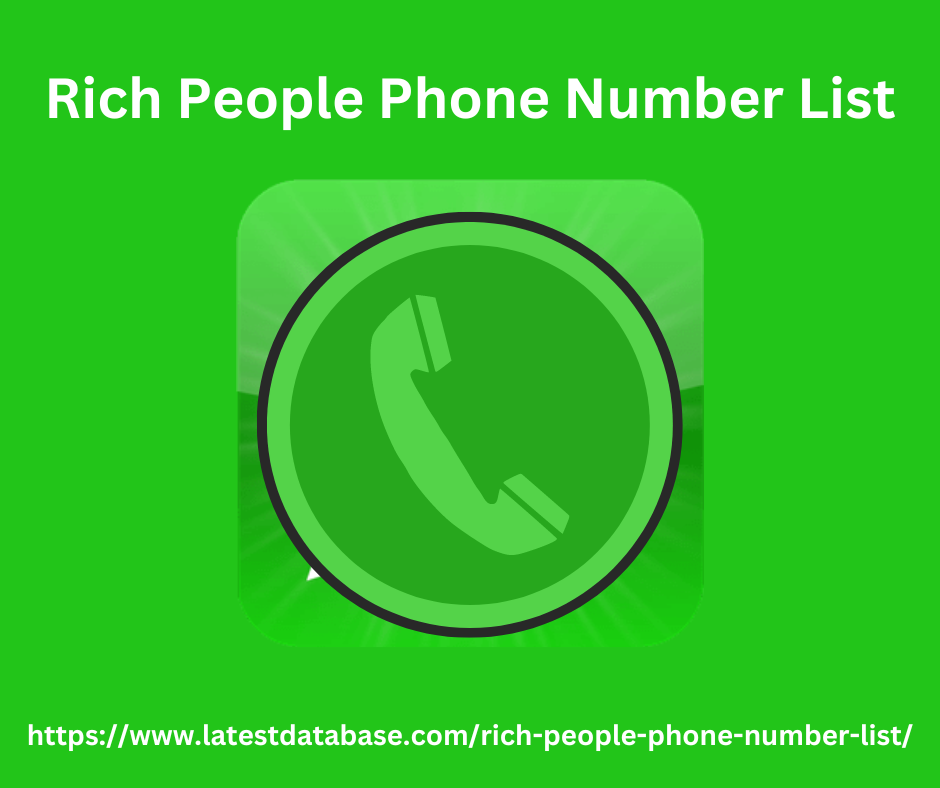
Improvement: Deep random forest, which introduces deep learning ideas based on traditional random forest, further improves the model's generalization ability and ability to handle complex patterns. The proposed extreme random forest X Lih optimizes the learning process of decision trees through the gradient boosting framework, greatly improving efficiency and accuracy. 4. Detailed explanation of the construction process of decision trees and random forests. Decision tree construction steps. Data preparation first preprocesses the data, including missing value filling, outlier processing, feature encoding and other operations. Feature selection calculates the information gain I or Gini of all features at each internal node. |
|